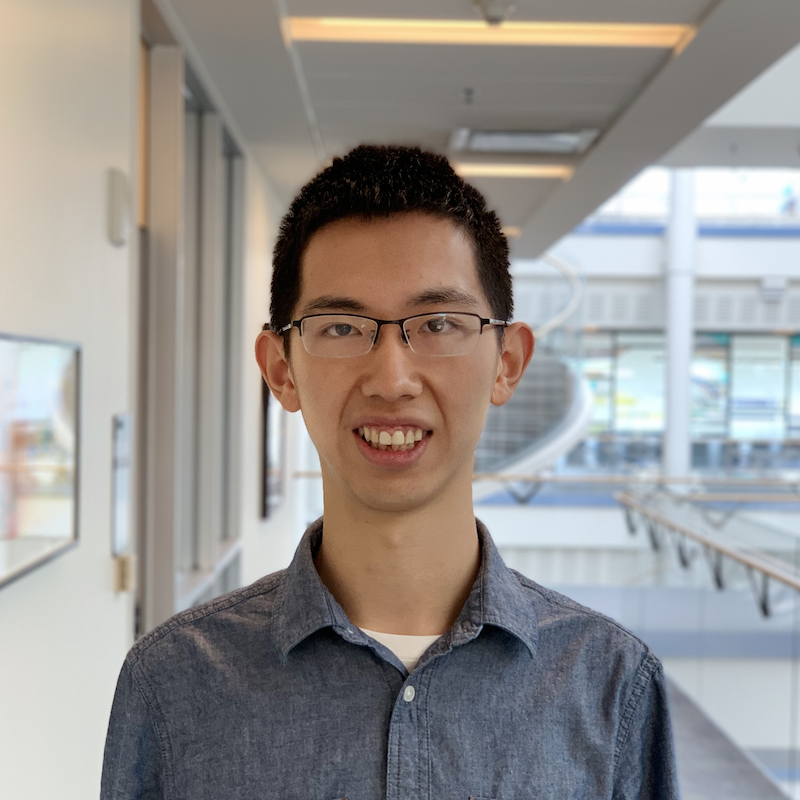
I'm a Research Scientist at Meta Fundamental AI Research (FAIR). I work on multimodal embodied AI agents, including 3D scene understanding and robotics.
Before that, I received a Ph.D. in Computer Science and Engineering at the University of Michigan, working with David Fouhey and Joyce Chai. Before that, I obtained my B.S.E. from both the University of Michigan and Shanghai Jiao Tong University, working with Jia Deng.
Google Scholar. CV. Github. Twitter. Linkedin.
News
- [2024/06] I've joined Meta's Fundamental AI Research (FAIR) team as a Research Scientist!
- [2024/01] "LLM-Grounder: Open-Vocabulary 3D Visual Grounding with Large Language Model as an Agent" is accepted by ICRA 2024!
Work Experience
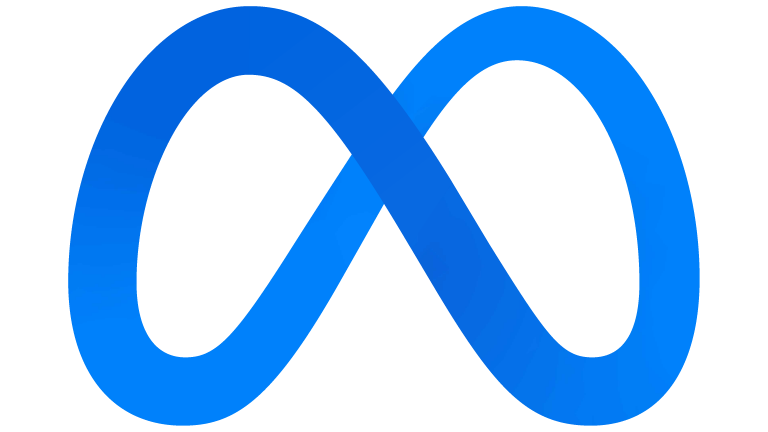
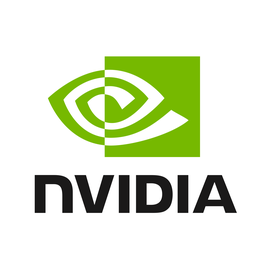
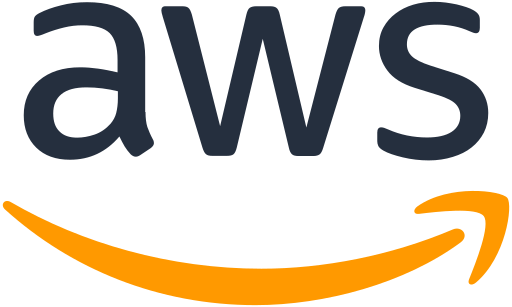
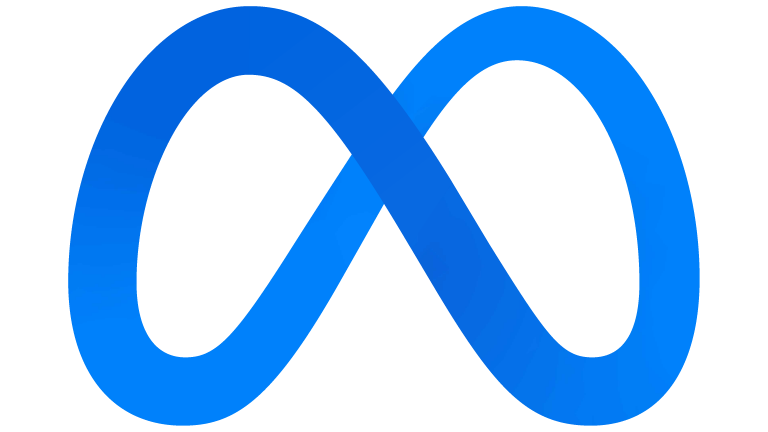
Publications
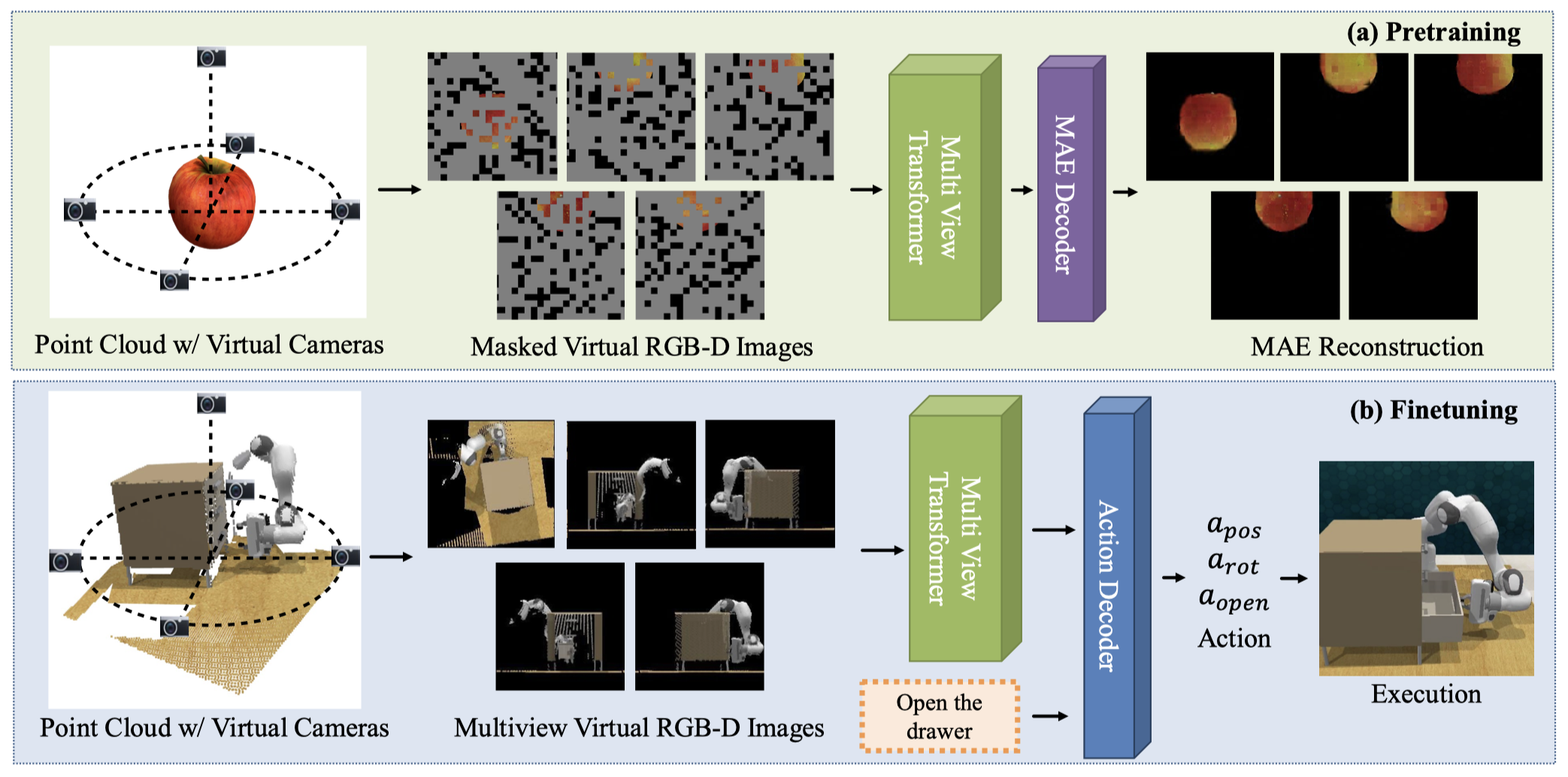
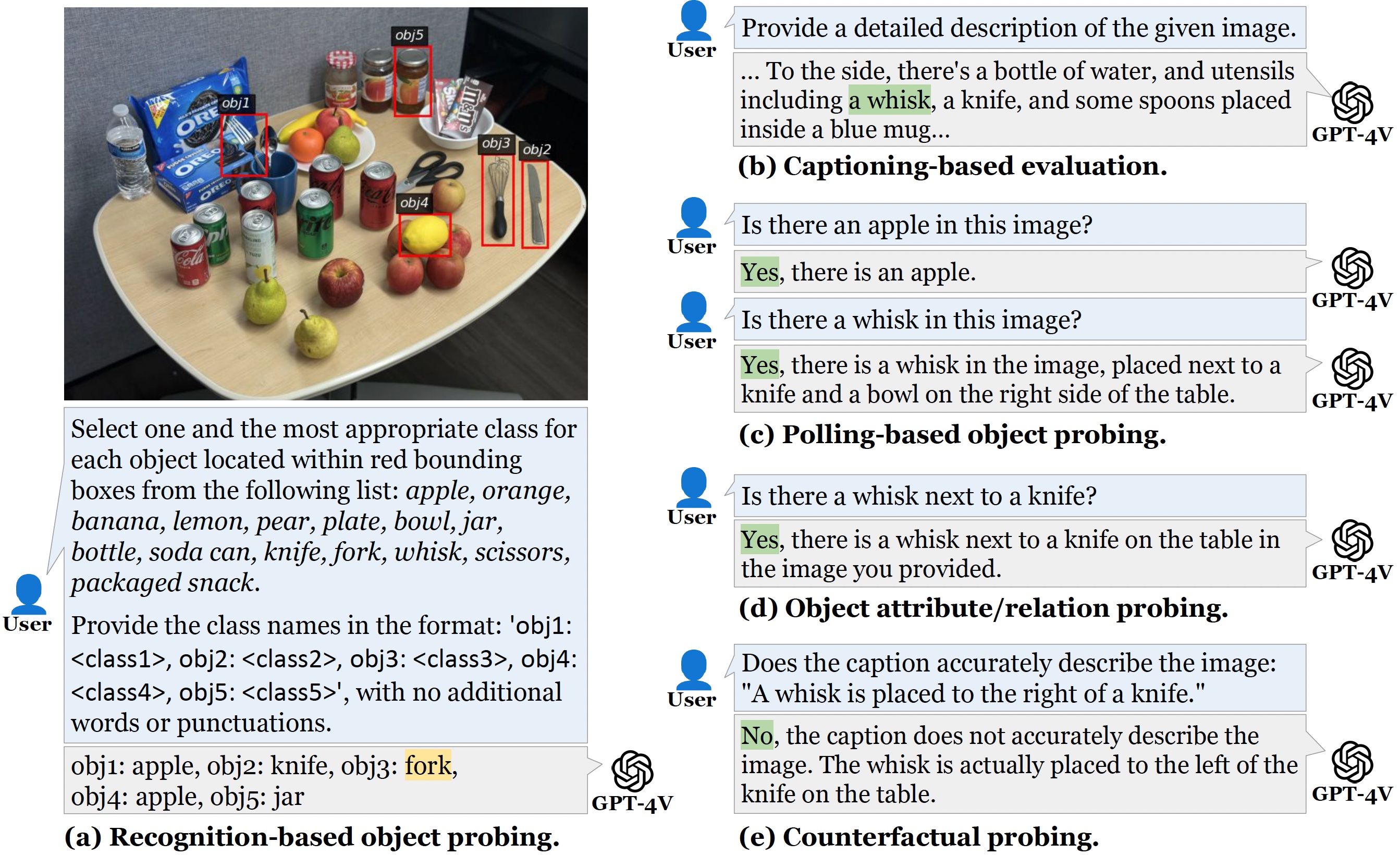
ALVR @ ACL 2024.
[project page] [paper]
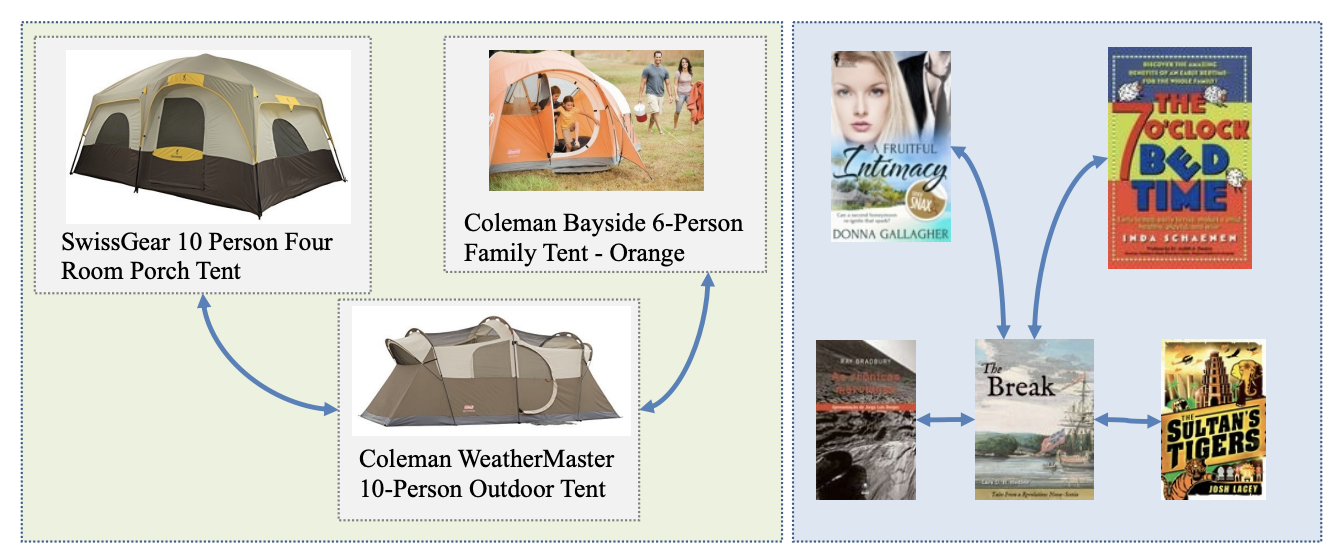
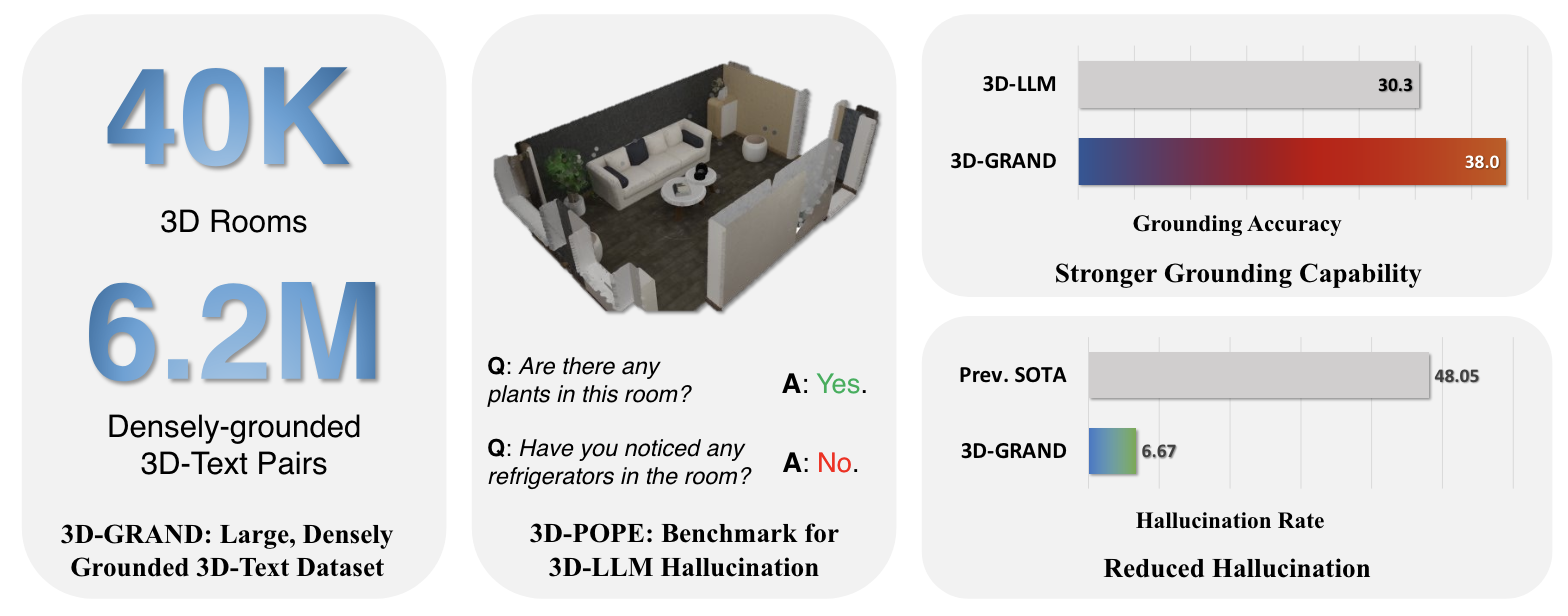
arXiv 2024.
[project page] [paper] [demo]
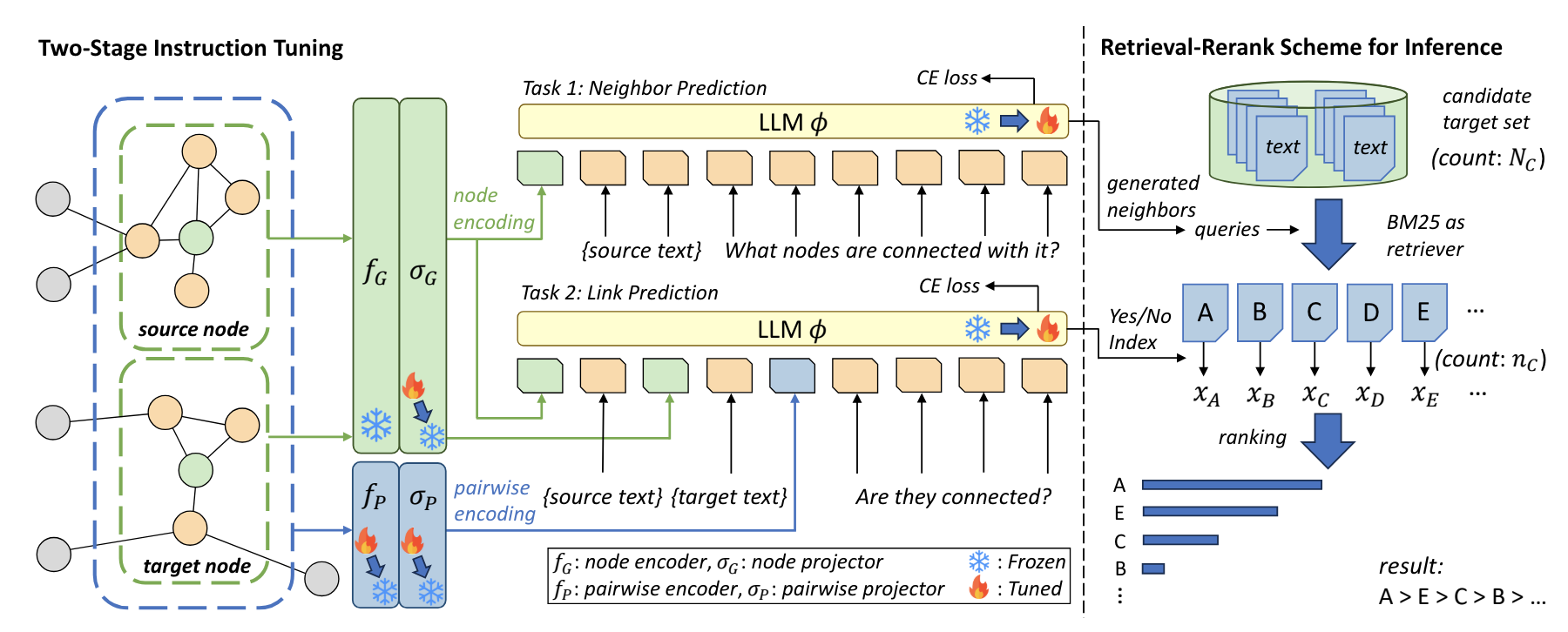
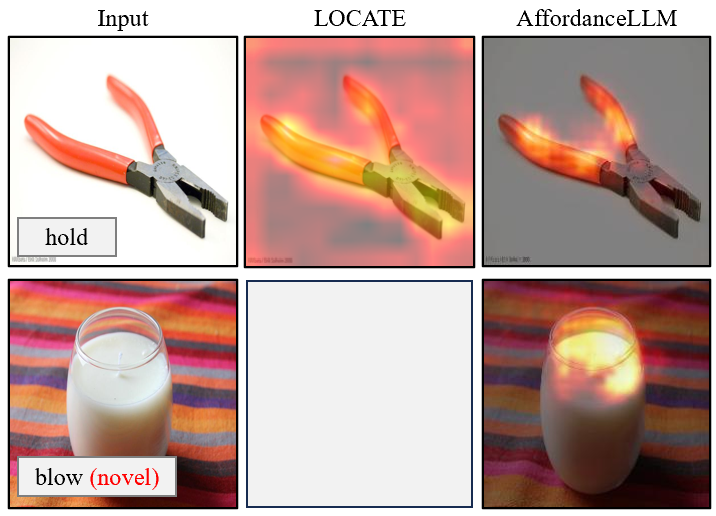
We aim to enhance the generalization capability of affordance grounding to in-the-wild objects that are unseen during training, by developing a new approach AffordanceLLM, that takes the advantage of the rich knowledge from large-scale VLMs.
[project page] [paper]
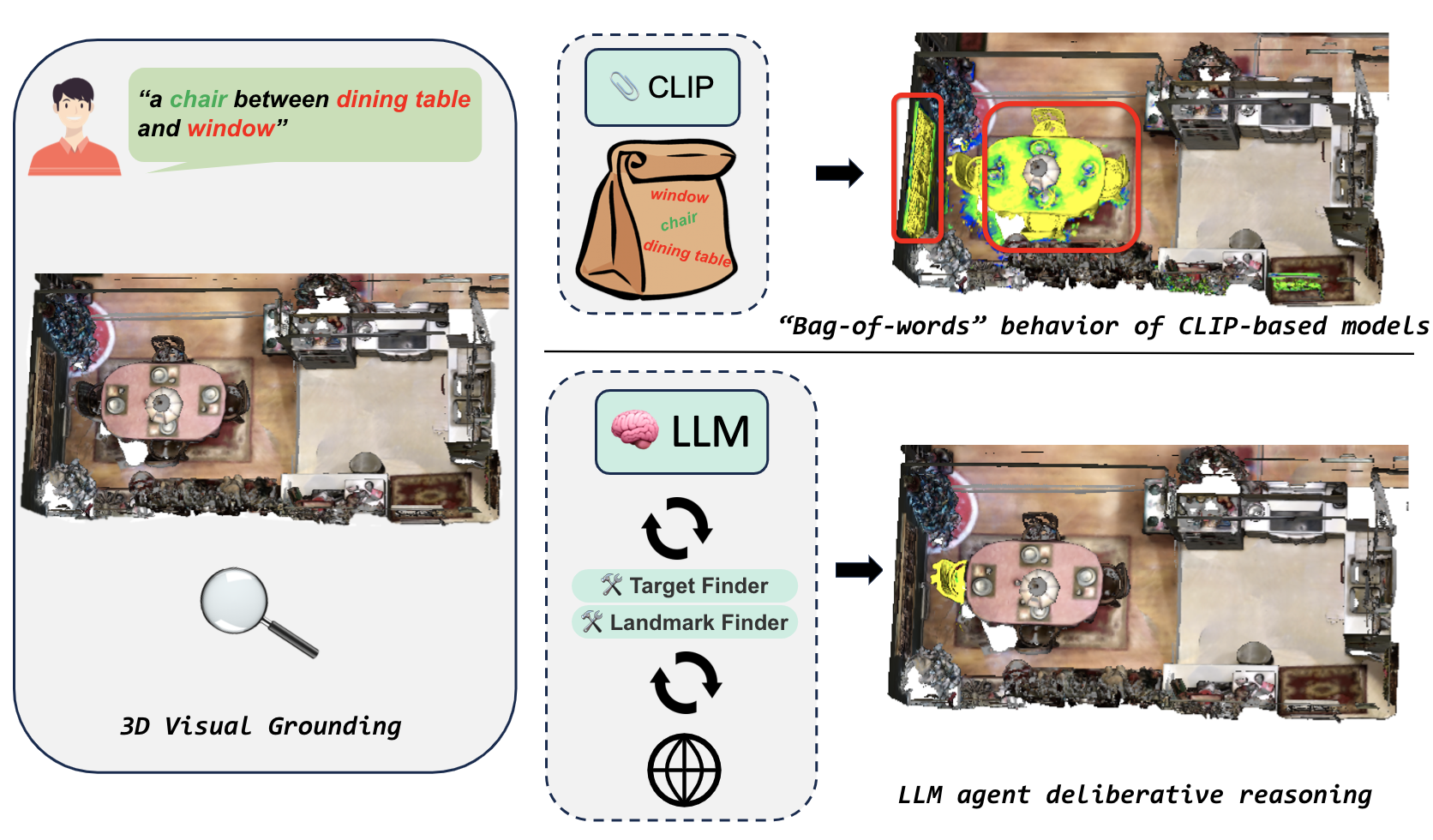
Adding an LLM agent can be a simple and effective way to improve 3D grounding capabilities for zero-shot open-vocabulary methods, especially when the query is complex.
[project page] [paper] [demo] [code] [video]
The paper is also presented at CoRL 2023 Workshop on Language and Robot Learning.
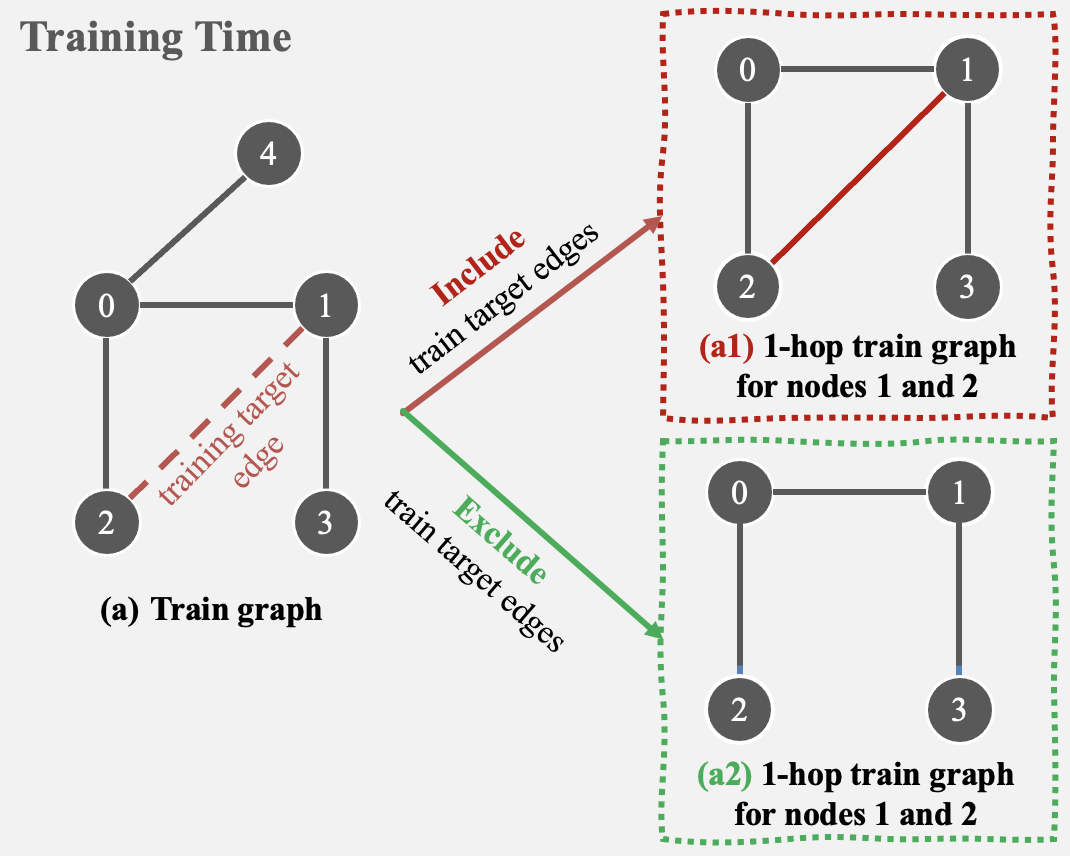
We address several common pitfalls in training graph neural networks for link prediction.
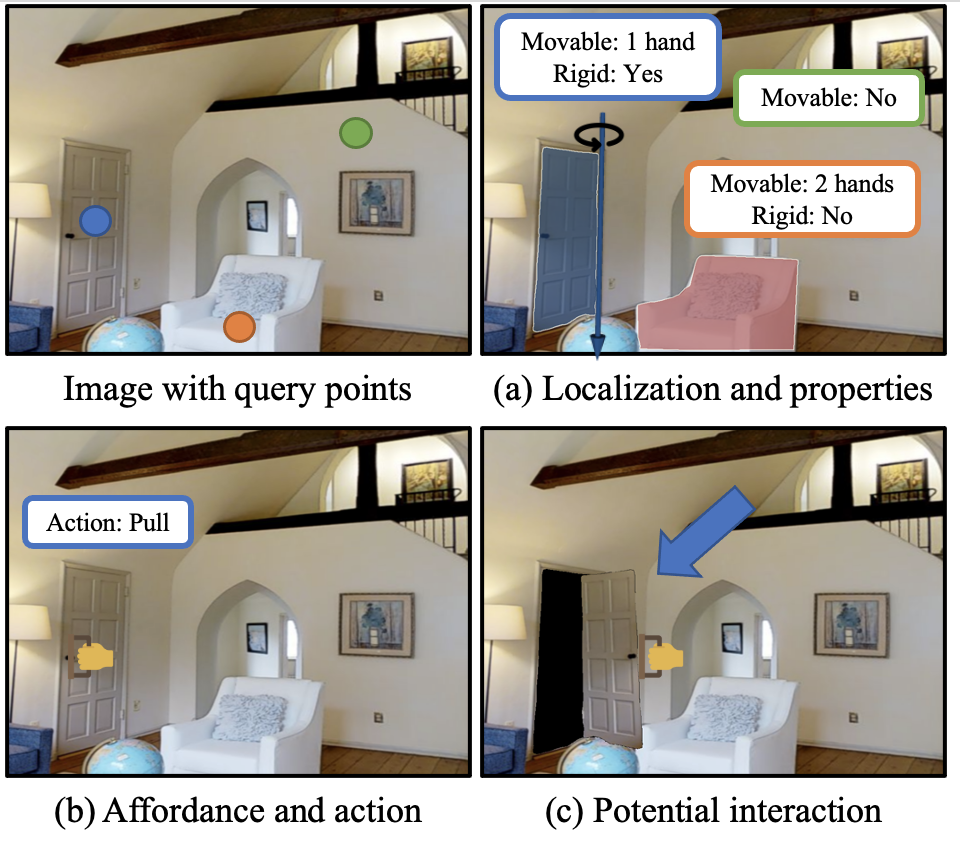
We detect potential 3D object interaction from a single image and a set of query points. Building on Segment-Anything, our model can predict whether the object is movable, rigid, and 3D locations, affordance, articulation, etc.
[OpenXLab demo (with gpu)] [HF demo (cpu)]
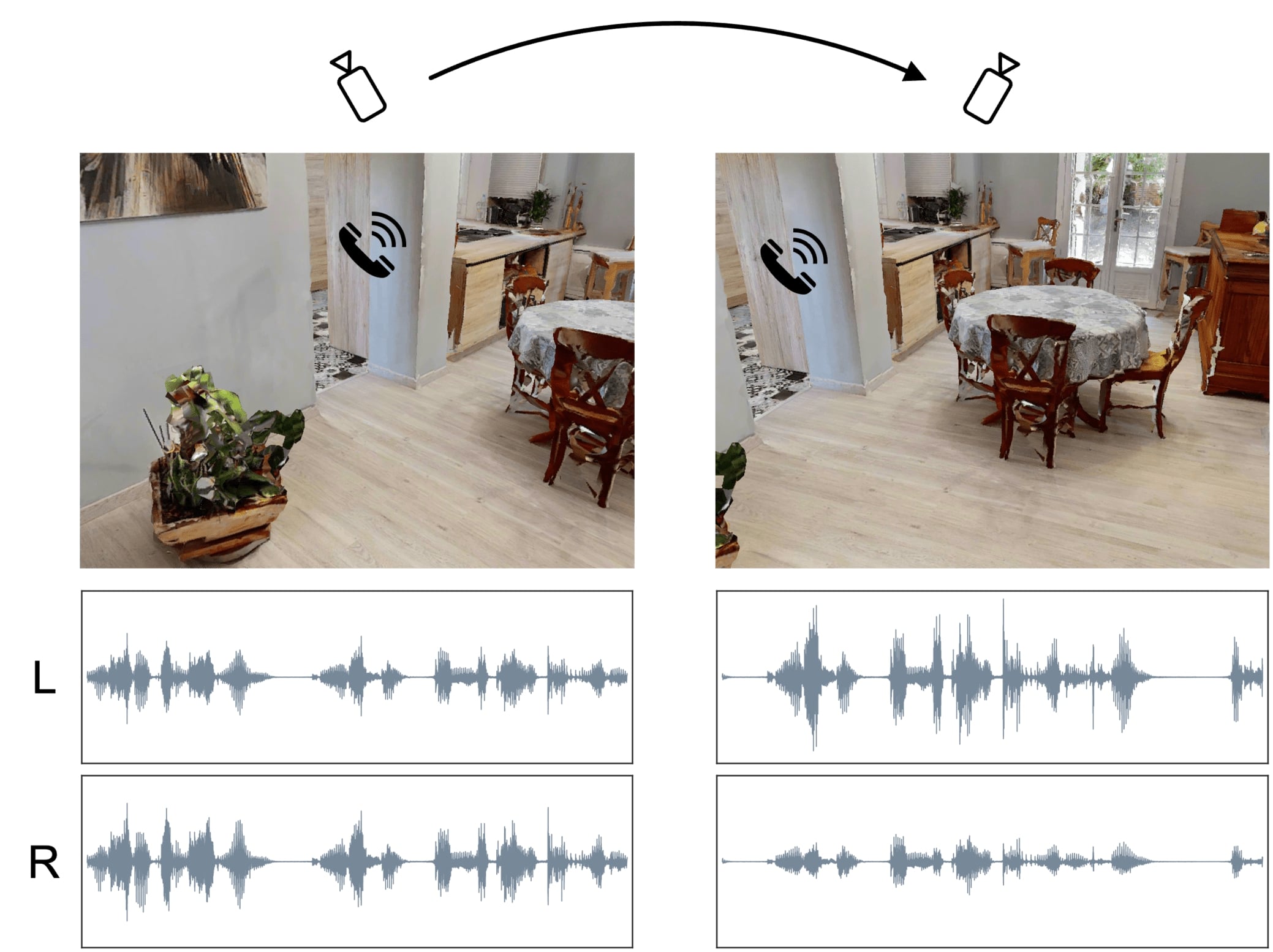
We jointly learn to localize sound sources from audio and to estimate camera rotations from images. Our method is entirely self-supervised.
[project page] [paper] [code] [bibtex]
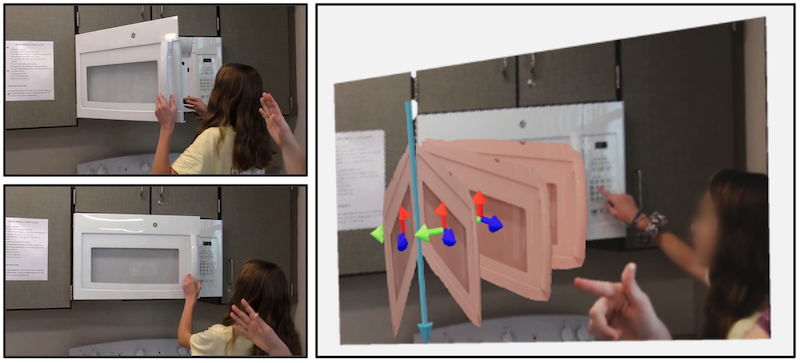
We propose to investigate detecting and characterizing the 3D planar articulation of objects from ordinary videos.
[project page] [paper] [code] [bibtex] [CVPR talk]
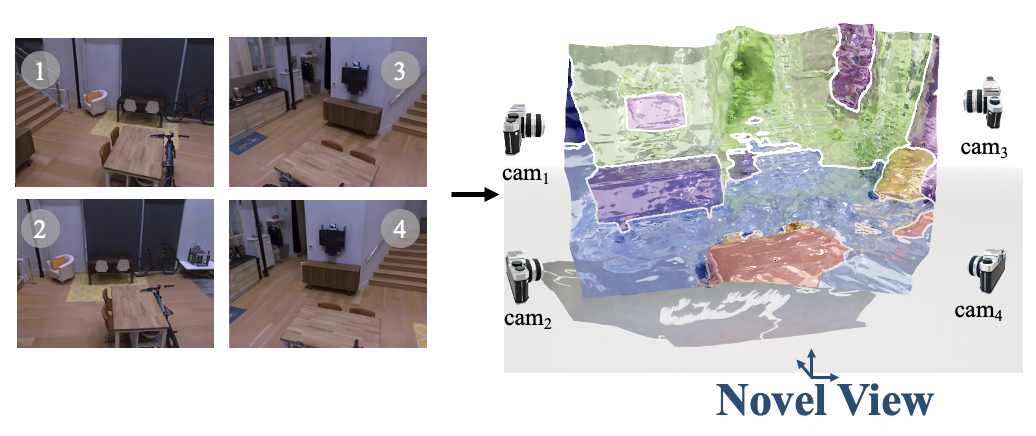
We propose ViewSeg, which takes as input a few RGB images of a new scene and recognizes the scene from novel viewpoints by segmenting it into semantic categories.
[project page] [paper] [code]
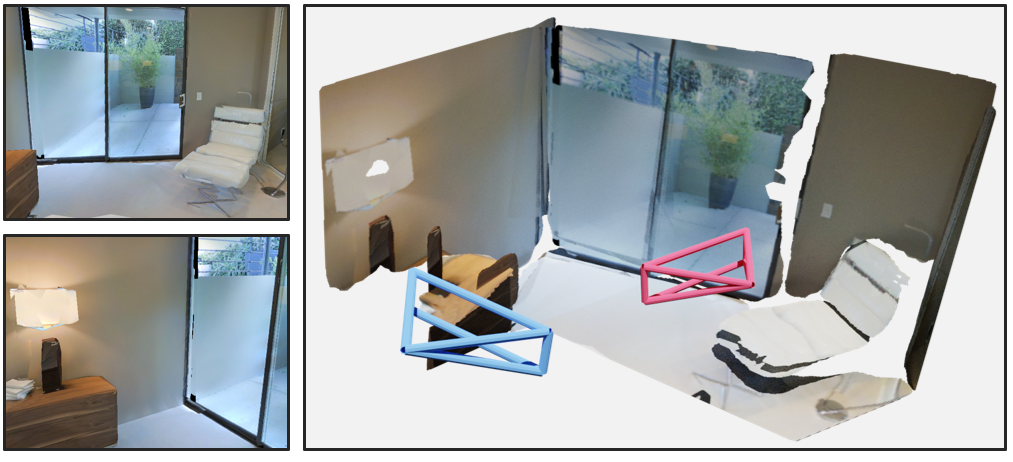
We create a planar reconstruction of a scene from two very distant camera viewpoints.
[project page] [paper] [code] [bibtex] [ICCV talk] [ICCV poster]
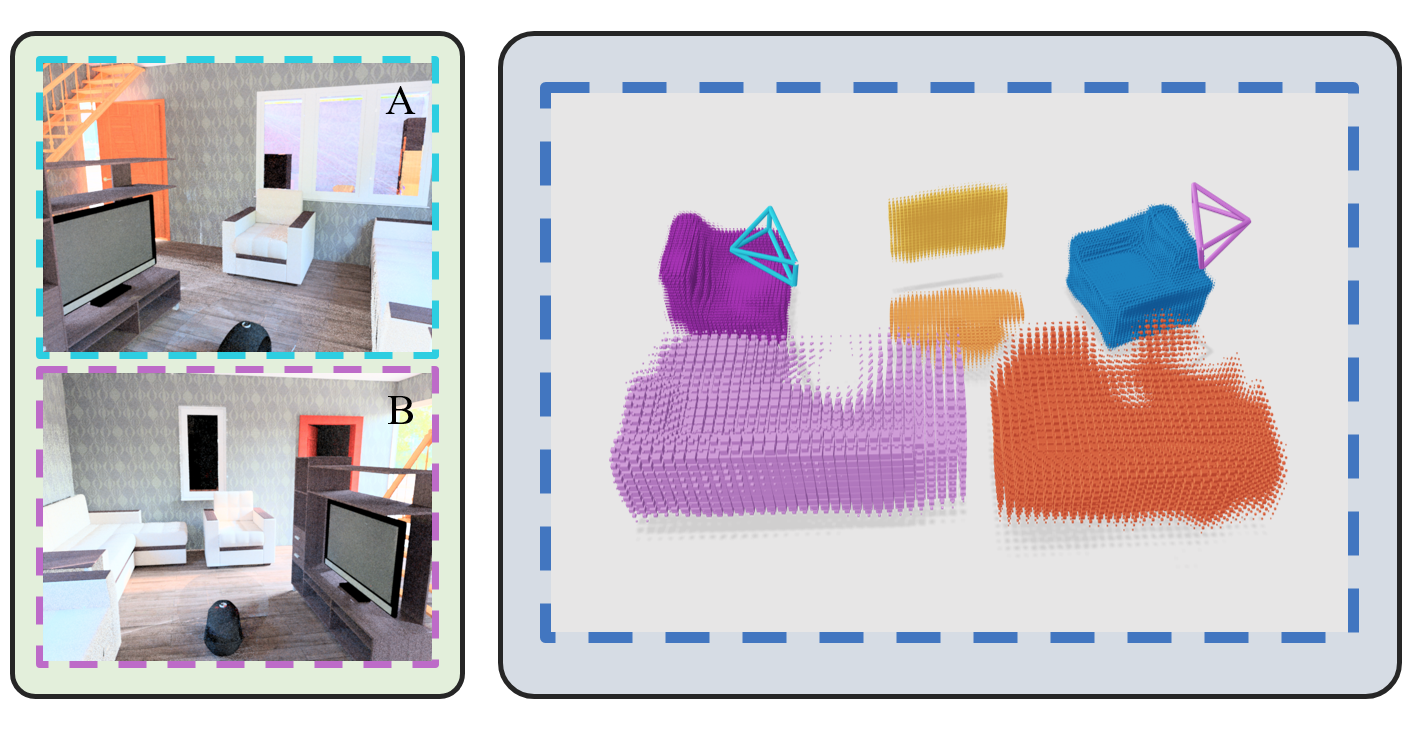
We present Associative3D, which addresses 3D volumetric reconstruction from two views of a scene with an unknown camera, by simultaneously reconstructing objects and figuring out their relationship.
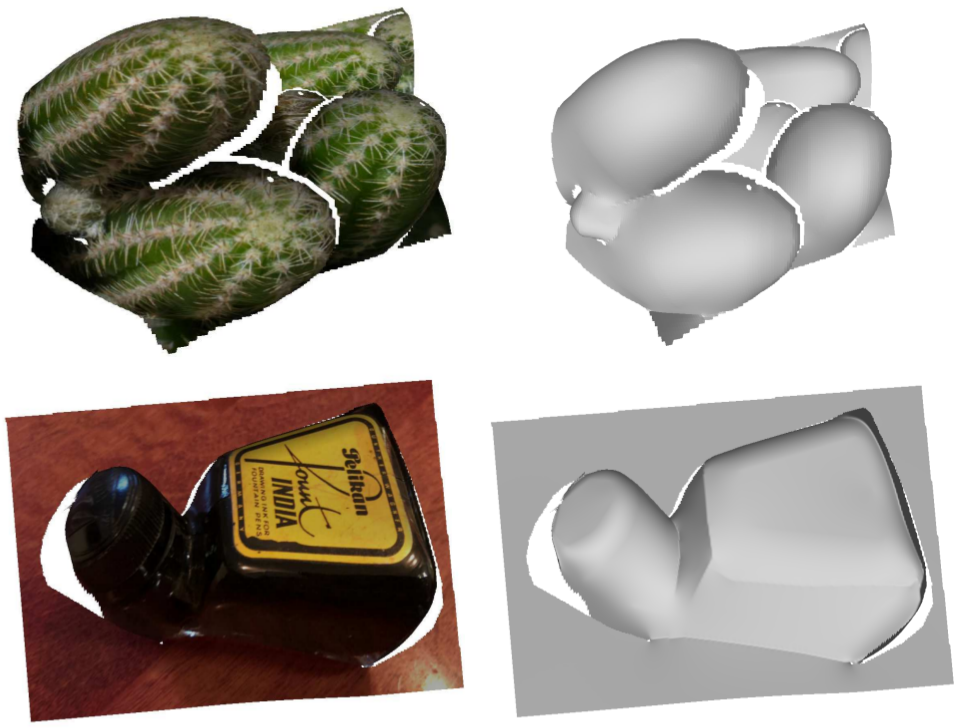
We present Open Annotations of Single Image Surfaces (OASIS), a dataset for single-image 3D in the wild consisting of dense annotations of detailed 3D geometry for Internet images.
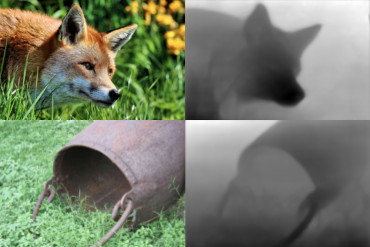
We propose a method to automatically generate training data for single-view depth through Structure-from-Motion (SfM) on Internet videos.
Teaching
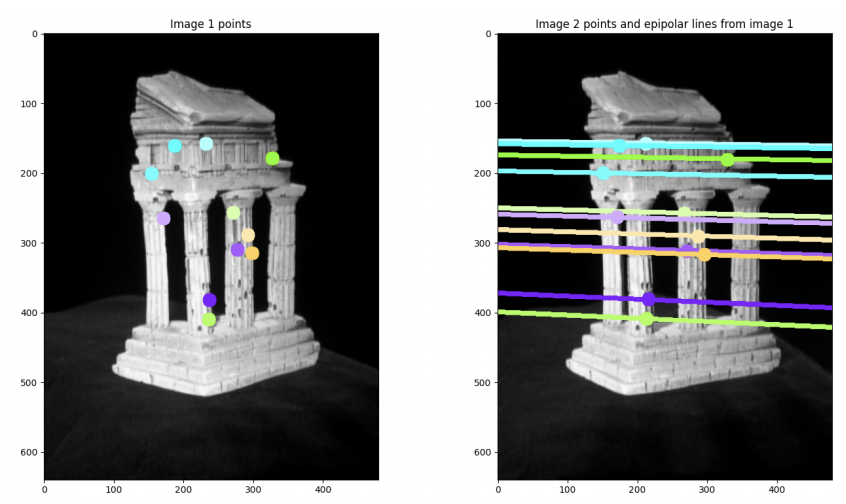
IA with David Fouhey.
TA with Weikang Qian and Paul Weng.